Vladimir Dvorkin
EECS Assistant Professor @UMich
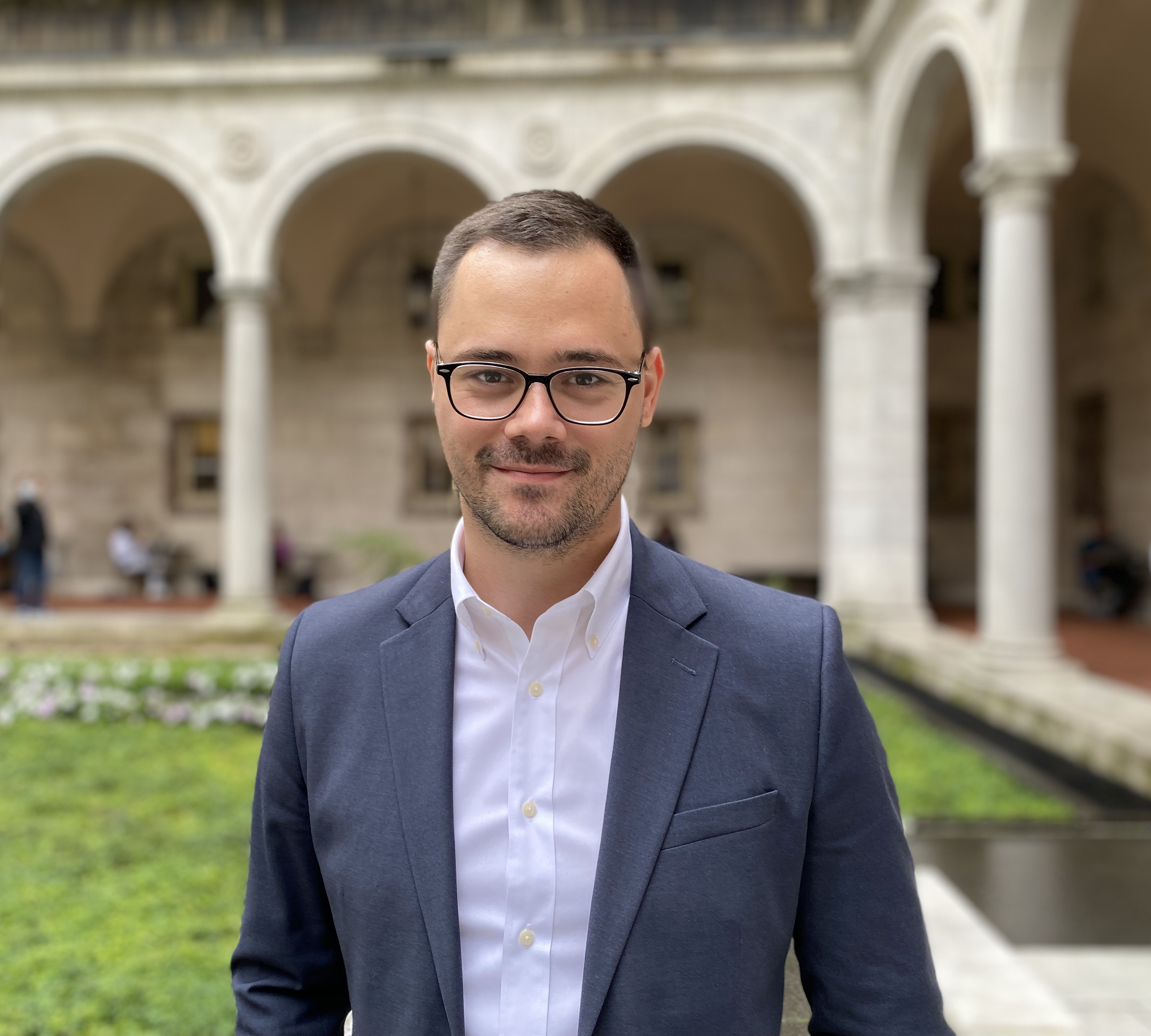
EECS 4240
1301 BEAL AVE, MI
Ann Arbor, MI 48109
I am an assistant professor of Electrical Engineering and Computer Science at the University of Michigan. I lead the ⏻ptiML group, where we harness the power of optimization and machine learning to address the grand challenges of transitioning to digital and carbon-free power and energy systems. The ⏻ptiML group specifically focuses on:
Prescriptive Energy Analytics: we develop prescriptive analytics at the crossroads of machine learning and optimization to support stochastic planning, operation and pricing in renewable-dominated systems.
Energy Data Privacy: we develop rigorous methods for the privacy-preserving utilization and sharing of energy data, treating privacy as an algorithmic notion, and improving transparency in the industry.
Trustworthy & Fair ML: we internalize physics and market constraints into machine learning models to ensure security, economic efficiency, and fairness in their applications to energy systems operations and markets.
Before joining University of Michigan, I was with the Laboratory for Information and Decision Systems (LIDS) and Energy Initiative of the Massachusetts Institute of Technology as a MSCA postdoctoral fellow. Before MIT, I graduated from the Technical University of Denmark and visited the School of Industrial and Systems Engineering of Georgia Tech. For more details, please see my CV.
News
- Nov 2024: Gave a CISE seminar at Boston University on the grid integration of AI under privacy and engineering constraints (slides).
- Spoke on the Nash regression equilibrium at the University of Michigan Control Seminars (slides, video).
- Oct 2024: Presented my work on harvesting data centers spatiotemporal flexibility for power systems at the 2024 IEEE CSS Day (slides, paper).
- Organized a session power systems on the road between optimization and learning at the 2024 INFORMS Annual Meeting.
- Sep 2024: Welcomed new PhD students Xinwei Lui, Shengyang Wu and Milad Hoseinpour to the group.
- Aug 2024: Excited to announce a new graduate course, Computational Power Systems, coming Winter 2025 (syllabus).
- AgentCONCUR—regression mechanism for data center coordination with power grids—is published in IEEE Transactions on Power Systems!
- Invited to the National Academies' workshop on Macroeconomic Implications of Decarbonization Policies and Actions in Washington DC.
- Jul 2024: Participated in the panel Decision-centric machine learning for power systems operation: Theory and application at the IEEE Power & Energy Society General Meeting in Seattle, Washington. Thanks to Yuanyuan Shi of UCSD for the invitation! (slides)
- Presented our work on trustworthy deep learning for electricity markets at the Federal Energy Regulatory Commission (recording)
- Presented Regression Equilibrium at the EURO conference in Copenhagen. Thanks to Lesia Mitridati of DTU for the invitation! (slides)
- May 2024: New preprint Regression equilibrium in electricity markets on market forces harmonizing private wind power forecasts
- Our new study on the offshore electricity market design has been accepted for publication in IEEE TEMPR (preprint, paper)
- Mar 2024: Presented our work on formal privacy guarantees in power systems at the Communication and Signal Processing seminar (video, slides)
- Jan 2024: Moved to Ann Arbor and started a new position as an Assistant Professor at the University of Michigan!
- Dec 2023: Our paper on the cost-optimal scheduling of renewables at the scale of the New York ISO has been published in IEEE TEMPR (paper)
- Nov 2023: Presented our work on price-aware predictions and fairness in electricity markets at the LIDS Climate Tea Talk at MIT (slides)
- Oct 2023: Price-aware deep learning is accepted at the NeurIPS 2023 Workshop on Tackling Climate Change with Machine Learning
- Presented our synthetic dataset generation algorithms for power systems at the 2023 INFORMS conference in Phoenix (slides)
- Sep 2023: New preprint Agent coordination via contextual regression (AgentCONCUR) for data center flexibility
- Aug 2023: New preprint Price-aware deep learning for electricity markets
- Jul 2023: Our work on aiding gas network optimization with input-convex neural networks is accepted at the CDC conference in Singapore
- Jun 2023: Our paper on synthetic dataset generation is accepted at the IEEE Control Systems Letters with the option to present at the CDC conference
- Presented our work on synthetic dataset generation at the Federal Energy Regulatory Commission (video)
- May 2023: Presented our work on gas network optimization with neural networks at the CCAI Workshop at the 2023 ICLR conference (video)
- Mar 2023: New preprint Differentially private algorithms for synthetic power system datasets
- Feb 2023: Our paper Multi-stage investment decision rules for power systems with performance guarantees is accepted for publication in IEEE TPWRS
- Sep 2022: New preprint Emission-constrained optimization of gas networks: Input-convex neural network approach
- New preprint Privacy-preserving convex optimization: When differential privacy meets stochastic programming
- Jun 2022: Presented our work on stochastic control of natural gas networks with linepack at the XXII PSCC conference in Porto (slides)