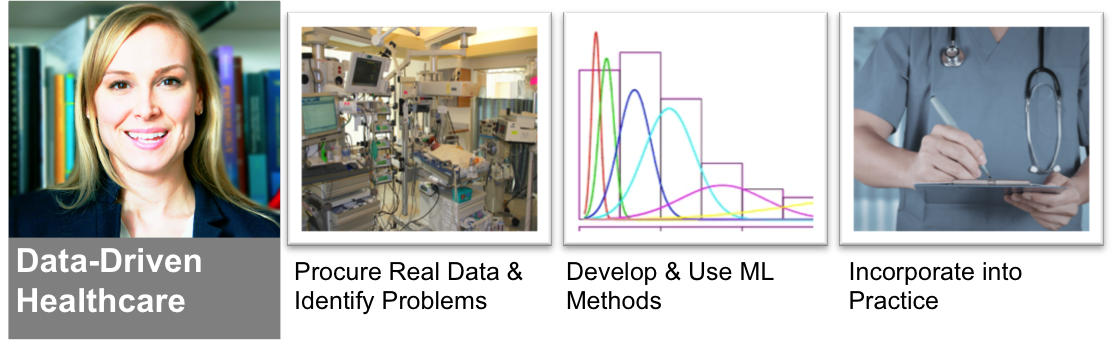
Research
OVERVIEW - Hospitals today are collecting an immense amount of patient data (e.g., images, lab tests, vital sign measurements), but are still ignoring the vast majority of it. Despite the fact that health data are messy and often incomplete, these data are useful and can help improve patient care. To this end, we have pioneered work in leveraging machine learning (ML) and electronic health records for predicting adverse outcomes or events (e.g., infections). Based on collaborations with 30+ clinicians, we have identified key characteristics for the safe and meaningful adoption of ML in healthcare [CID'17 ]. Beyond accuracy, models must be, actionable (tell a clinician how to reduce a patient’s risk not just who’s at risk) and robust (capable of adapting to changes across populations and time). Achieving accurate models with these characteristics presents unique technical challenges. Specifically, in healthcare, one often deals with high-dimensional data (i.e., many covariates) but has few examples to learn from –‘high D small N.’ To address these challenges, we have developed new ML techniques.
METHODOLOGICAL CONTRIBUTIONS
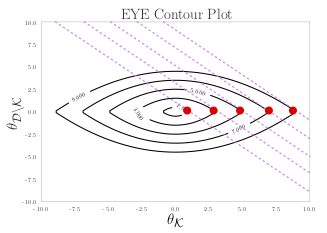
Incorporating Domain Expertise - recently, we proposed a new regularization penalty, the EYE penalty, that incorporates domain knowledge regarding known risk factors. In ML, and specifically deep learning, domain knowledge is oftentimes ignored. However, in healthcare, domain knowledge can be critical when training data are limited. Our proposed approach uses domain knowledge to help select among highly correlated variables. This leads to more robust models, by reducing the effects of confounding [KDD'18a ]. This work is supported by an NSF CAREER Award, and has garnered attention from other domains where models should agree, at least in part, with domain knowledge. In addition, we have shown how domain knowledge can help in designing network architectures to exploit domain specific-invariances. Invariances are transformations that when applied to the input do not affect the output. Such transformations are often domain or task specific. E.g., many of the architectures proposed in computer vision are designed to efficiently exploit invariances that arise in tasks involving natural images (e.g., translation invariance). Using domain knowledge about the presence/absence of invariances in tasks involving medical images, we proposed two new CNN architecture modifications tailored to brain images [MLHC'18a ]. In contrast to a standard CNN, in which the same filter/feature is convolved over the entire image, our approach learns region-specific features, since a pattern may have a different meaning depending on where in the brain it arises.
Sequence Transformer Networks - When training data are limited, ML practitioners can augment their training data by transforming their data in such a way that invariances are exploited e.g., cropping, flipping, or rotating an image. However, given heterogeneous clinical time-series data, data augmentation is not straightforward. Still, we expect certain temporal invariances to arise in tasks involving clinical time-series data, since oftentimes the relative ordering of events is more important than their precise timing. This intra-class variation can be addressed using techniques like dynamic time warping, but at inference time such algorithms are slow. With the goal of speeding up inference, while exploiting task-specific temporal invariances, we developed techniques for automatically learning invariances. Our proposed approach, sequence transformer networks (STNs), learns to transform clinical time-series data, so as to minimize intra-class variations [MLHC'18b ]. Applied to the task of predicting in-hospital mortality, this led to improvements in predictive performance over state-of-the-art.
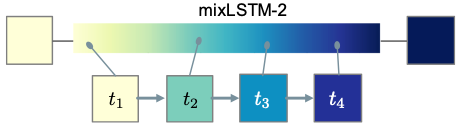
Relaxed Parameter Sharing over Time - In addition, to exploiting invariances, it is important to recognize what invariances are not present in a task. Specifically, we have identified temporal shift in many healthcare tasks, in which the relationship between the input and the output changes over time (e.g., when risk factors change over time). Accurately modeling these time-varying relationships is especially difficult with limited training data. Thus, we recently proposed a new approach for relaxed weight sharing, mixLSTM. Our approach learns multiple sets of parameters and how to combine these parameters at each time step. This is more parameter efficient compared to simply learning different parameters at each time step, and more flexible than a recurrent neural network structure in which parameters are shared across all time steps. Applied to three clinically relevant in-patient prediction tasks, the proposed approach led to significant improvements over several state-of-the-art baselines [MLHC'19 ].
APPLICATIONS
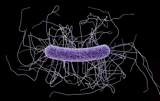
Learning to Prevent Healthcare-Associated Infections - Healthcare-acquired infections are associated with significant morbidity. In our work on predicting patient risk of infection, we i) pose the problem as a high-dimensional time-series classification task, developing techniques that account for changes over time [ NeurIPS'12, OFID'14, JMLR'16], and ii) established the importance of hospital-specific models and transfer learning techniques [ JAMIA'14, ICHE'18]. This work has led to the development of accurate models for identifying patients at risk of C. difficile infection. We have validated the results at six separate hospitals and have shown how a similar approach can be used to predict other outcomes [OFID'19 ]. In addition, we have developed techniques that can account for asymptomatic colonized patients, who may unknowingly spread disease [AAAI'18 ]. The work described above, culminated in a model tailored to Michigan Medicine (UM) that is currently being applied to daily streams of data to calculate the daily risk of infection of UM inpatients.
Learning from Physiological Signals with Applications in Type I Diabetes - To manage blood glucose levels, individuals with type 1 diabetes (T1D) must constantly make decisions about their regimen. To alleviate this decision fatigue, we are working on novel prediction and control techniques that aim to accurately predict glucose values and the amount of required insulin. More specifically, when learning to predict hyper- and hypoglycemic events, we have shown i) how jointly modeling patterns in the data and the context under which those patterns occur improves performance [KDD'17 ] and ii) that when forecasting blood glucose values, architectures that automatically encode temporal dependencies, while constraining the output, can lead to more accurate forecasts [KDD'18b ]. Currently, we are developing reinforcement learning techniques to learn policies for controlling blood glucose [ICML-WKSP'19 ]. We are now working with JDRF and endocrinologists to translate this work to tools that can improve the lives of people with T1D.
Preprints/Publications/Presentations
- Ian Fox, and Jenna Wiens Advocacy Learning: Learning through Competition and Class-Conditional Representations, IJCAI, 2019.
- Jeeheh Oh, Jiaxuan Wang, Shengpu Tang, Michael Sjoding, and Jenna Wiens, Relaxed Weight Sharing: Effectively Modeling Time-Varying Relationships in Clinical Time-Series, MLHC, 2019.
- Michael Sjoding, Shengpu Tang et al., Democratizing EHR Analyses a Comprehensive Pipeline for Learning from Clinical Data, MLHC (Clinical Abstract), 2019 [to appear].
- Erkin Otles, Haozhu Wang, et al., Return to Work After Injury: A Sequential Prediction and Prescription Problem, MLHC (Clinical Abstract), 2019 [to appear].
- Donna Tjandra, Raymond Migrino, Bruno Giordani, and Jenna Wiens, An EHR-based Cohort Discovery Tool for Identifying Probable AD, Alzheimer's Association International Conference (AAIC), 2019.
- Donna Tjandra, Raymond Migrino, Bruno Giordani, and Jenna Wiens, EHR-based Patient Risk Stratification Tool for Probable AD, Alzheimer's Association International Conference (AAIC), 2019.
- Ian Fox and Jenna Wiens, Reinforcement Learning for Blood Glucose Control: Challenges and Opportunities, RL4RealLife Workshop, ICML, 2019.
- Ben Li, Jeeheh Oh, Vincent Young, Krishna Rao, and Jenna Wiens, Using Machine Learning and the Electronic Health Record to Predict Complicated Clostridium difficile Infection, Open Forum Infectious Diseases 6(5), 2019.
- Daniel Zeiberg, Tejas Prahlad, Brahmajee Nallamothu, Theordore J. Iwashyna, Jenna Wiens* and Michael Sjoding*, Machine learning for patient risk stratification for acute respiratory distress syndrome, PLOS ONE 14 (13), 2019. *co-senior authors
- Tian Bao, Brooke N. Klatt, Susan L. Whitney, Kathleen H. Sienko, and Jenna Wiens Automatically evaluating balance: a machine learning approach, IEEE Transactions on Neural Systems and Rehabilitation Engineering, 2019.
- Saige Rutherford, Pascal Sturmfels et al., Observing the origins of human brain development: Automated processing of fetal fMRI, bioRxiv, 2019.
- Devendra Goyal, Donna Tjandra, et al., Characterizing heterogeneity in the progression of Alzheimer's disease using longitudinal clinical and neuroimaging biomarkers, Alzheimer's & Dementia: Diagnosis, Assessment & Disease Monitoring, 2018.
- Jeeheh Oh, Jiaxuan Wang, and Jenna Wiens Learning to Exploit Invariances in Clinical Time-Series Data using Sequence Transformer Networks, MLHC, 2018.
- Pascal Sturmfels et al., A Domain Guided CNN Architecture for Predicting Age from Structural Brain Images, MLHC, 2018.
- Jenna Wiens and James Fackler, Striking the Right Balance - Applying Machine Learning to Pediatric Critical Care Data, Pediatric Critical Care Medicine, 2018.
- Ian Fox et al., Deep Multi-Output Forecasting: Learning to Accurately Predict Blood Glucose Trajectories, KDD, August 2018.
- Jiaxuan Wang, et al., Learning Credible Models, KDD, August 2018.
- Jeeheh Oh, Maggie Makar et al., A Generalizable, Data-Driven Approach to Predict Daily Risk of Clostridium difficile Infection at Two Large Academic Health Centers, Infection Control and Hospital Epidemiology, March 2018.
- Devendra Goyal, Zeeshan Syed, and Jenna Wiens, Clinically Meaningful Comparisons Over Time: An Approach to Measuring Patient Similarity based on Subsequence Alignment, arXiv:1803.00744, 2018.
- Jiaxuan Wang, Ian Fox, Jonathan Skaza, Nick Linck, Satinder Singh, and Jenna Wiens, The Advantage of Doubling: A Deep Reinforcement Learning Approach to Studying the Double Team in the NBA, Sloan Sports Analytics Conference, February 2018. [Poster]
- Maggie Makar, John Guttag, and Jenna Wiens, Learning the Probability of Activation in the Presence of Latent Spreaders, AAAI, February 2018. (oral presentation)
- Jenna Wiens, Graham Snyder et al., Potential Adverse Effects of Broad-Spectrum Antimicrobial Exposure in the Intensive Care Unit, Open Forum Infectious Diseases, December 2017.
- Eli Sherman et al., Leveraging Clinical Time-Series Data for Prediction: A Cautionary Tale, AMIA Annual Symposium, November 2017. (oral presentation)
- Jeeheh Oh, Maggie Makar et al., A Data-Driven Approach to Predict Daily risk of Clostridium difficile Infection at Two Large Academic Health Centers, Infectious Disease Week, October 2017.
- Jenna Wiens and Erica Shenoy, Machine Learning for Healthcare: On the Verge of a Major Shift in Healthcare Epidemiology, Clinical Infectious Diseases, August 2017.
- Ian Fox et al., Contextual Motifs: Increasing the Utility of Motifs using Contextual Data, KDD, August 2017. (Oral Presentation Acceptance Rate: 8.5%)
- Jose Javier Gonzalez Ortiz, Cheng Perng Phoo, and Jenna Wiens, Heart Sound Classification Based on Temporal Alignment Techniques, Computing in Cardiology, September 2016.[Code: link]
- Mason Wright and Jenna Wiens, Method to their March Madness: Insights from Mining a Novel Large-Scale Dataset of Pool Brackets, KDD Workshop on Large-Scale Sports Analytics, August 2016.
- Jenna Wiens, John Guttag, and Eric Horvitz, Patient Risk Stratification with Time-Varying Parameters: A Multitask Learning Approach, JMLR, April 2016.
- Avery McIntyre et al., Recognizing and Analyzing Ball Screen Defense in the NBA, Sloan Sports Analytics Conference, March 2016.[Slides: pdf]
- Abhishek Bafna and Jenna Wiens, Automated Feature Learning: Mining Unstructured Data for Useful Abstractions, ICDM, November 2015.
- Abhishek Bafna and Jenna Wiens, Learning Useful Abstractions from the Web , AMIA Annual Symposium, November 2015. (poster)
- Sai R. Gouravajhala et al., An LED Blink is Worth a Thousand Packets: Inferring a Networked Device's Activity from its LED Blinks, USENIX Summit on Information Technologies for Health, August 2015.
- Devendra Goyal, Zeeshan Syed, and Jenna Wiens, Predicting Disease Progression in Alzheimer's Disease, MUCMD, August 2015.
- Jenna Wiens et al., Learning Data-Driven Patient Risk Stratification Models for Clostridium difficile,Open Forum Infectious Diseases, July 2014.
- Jenna Wiens, Learning to Prevent Healthcare-Associated Infections: Leveraging Data Across Time and Space to Improve Local Predictions, PhD Thesis, MIT, May 2014.
- Jenna Wiens et al., Automatically Recognizing On-Ball Screens, Sloan Sports Analytics Conference, Feb 2014.
- Jenna Wiens et al., A Study in Transfer Learning: Leveraging Data from Multiple Hospitals to Enhance Hospital-Specific Predictions, Journal of the American Medical Informatics Association, Jan 2014.
- Jenna Wiens et al., To Crash or Not to Crash: A quantitative look a the relationship between offensive rebounding and transition defense in the NBA, Sloan Sports Analytics Conference, March 2013.
- Jenna Wiens et al., Patient Risk Stratification for Hospital-Associated C. diff as a Time-Series Classification Task, Neural Information Processing Systems (NIPS), Dec 2012. [Video]
- Jenna Wiens et al., Learning Evolving Patient Risk Processes for C. diff Colonization, ICML Workshop on Clinical Data Analysis, June 2012.[Slides: pdf]
- Jenna Wiens et al., On the Promise of Topic Models for Abstracting Complex Medical Data: A Study of Patients and their Medications, NIPS Workshop on Personalized Medicine, December 2011.
- Jenna Wiens and John Guttag, Patient-Specific Ventricular Beat Classification without Patient-Specific Expert Knowledge: A Transfer Learning Approach, IEEE EMBS Conference, September 2011.
- Jenna Wiens and John Guttag, Active Learning Applied to Patient-Adaptive Heartbeat Classification, Neural Information Processing Systems (NIPS), December 2010.
- Jenna Wiens, Machine Learning for Ectopic Beat Classification, Master's thesis, MIT, May 2010.
- Jenna Wiens and John Guttag, Patient-Adaptive Ectopic Beat Classification using Active Learning. , Computing in Cardiology (CinC) September 2010. [Slides: pptx]
Multimedia
Women in Tech Shown | Scientific American | ACP Hospitalist Article | MIT Tech Review Article | SSAC16 | Invited Talk at Wellesley College: Big Data's Impact in Medicine, Finance, and Sports | SSAC13: To Crash or not to Crash | ESPN TrueHoop TV: Interview with Henry Abbott | ESPN TrueHoop: Commentary | Grantland Interview | NIPS 2012 Spotlight | NIPS Workshops 2011 Spotlight